Data Science Technique
In the realm of data science techniques, the explosion of data has presented both opportunities and challenges. With the advent of big data, datasets are becoming increasingly complex and high-dimensional, posing significant computational and analytical hurdles. However, dimensionality reduction techniques offer a powerful solution to tackle these challenges, enabling data scientists to extract meaningful insights from large, intricate datasets efficiently.
Dimensionality reduction refers to the process of reducing the number of variables or features in a dataset while preserving its essential characteristics. By eliminating redundant or irrelevant features, dimensionality reduction techniques aim to simplify the dataset’s structure, making it more manageable and interpretable without sacrificing crucial information.
The data science techniques play a crucial role in various applications, including pattern recognition, classification, clustering, and visualization.
One of the most widely used dimensionality reduction in data science techniques is Principal Component Analysis (PCA). PCA seeks to transform high-dimensional data into a lower-dimensional space by identifying the principal components that capture the maximum variance in the dataset. Let’s delve into the implementation of PCA using Python to illustrate its effectiveness in reducing dimensionality.
In this example, we applied PCA in data science technique to the Iris dataset—a classic benchmark dataset in machine learning. By reducing the dimensionality of the dataset from four features to two principal components, we were able to visualize the data in a two-dimensional space while preserving most of the original variance.
Another dimensionality reduction technique worth mentioning is t-distributed Stochastic Neighbor Embedding (t-SNE). Unlike PCA, which focuses on preserving global structure, t-SNE aims to preserve local structure, making it particularly useful for visualizing high-dimensional data in low-dimensional space. Let’s explore how to implement t-SNE using Python.
In this example, we applied t-SNE to the same Iris dataset to visualize it in a two-dimensional space. The resulting plot highlights the clusters formed by different classes of iris flowers, demonstrating the effectiveness of t-SNE in capturing the underlying structure of high-dimensional data.Suppose we have a dataset containing gene expression profiles of tumor samples from breast cancer patients. Each sample in the dataset represents the expression levels of thousands of genes.
Our goal is to classify the tumor samples into different subtypes of breast cancer (e.g., luminal A, luminal B, HER2-enriched, basal-like) based on their gene expression profiles.We can use dimensionality reduction techniques, such as PCA, to extract the most informative features (genes) from the high-dimensional gene expression data and visualize the samples in a lower-dimensional space.Here’s how we can do it:
-
Data Preprocessing: We preprocess the gene expression data by normalizing the expression levels and handling missing values if any. Dimensionality Reduction: We apply PCA to reduce the dimensionality of the data. PCA will identify the principal components (PCs) that capture the most variation in the data.
Visualization: We visualize the samples in a two-dimensional or three-dimensional space using the first two or three principal components as axes. Each sample is represented as a point in the plot. Classification: We can then use machine learning algorithms, such as logistic regression or support vector machines, to classify the tumor samples based on their reduced-dimensional representation. Evaluation: We evaluate the performance of the classification model using metrics such as accuracy, precision, recall, and F1-score.
By visualizing the samples in a reduced-dimensional space, we can gain insights into the underlying structure of the data and potentially discover patterns or clusters corresponding to different subtypes of breast cancer. This can aid in both exploratory data analysis and building predictive models for cancer diagnosis and treatment.
Dimensionality reduction techniques like PCA and t-SNE offer invaluable insights into complex datasets, enabling data scientists to explore, analyze, and visualize high-dimensional data effectively.
By embracing these techniques, data scientists can uncover hidden patterns, reduce computational complexity, and make informed decisions based on a simplified representation of the data. As the volume and complexity of data continue to grow, dimensionality reduction techniques will remain indispensable tools in the data scientist’s toolkit, empowering them to navigate the intricacies of high-dimensional data analysis with confidence and precision.
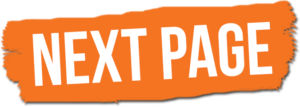
https://www.scholarnews.online